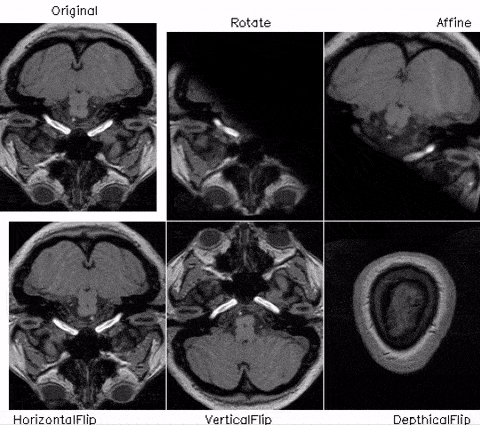
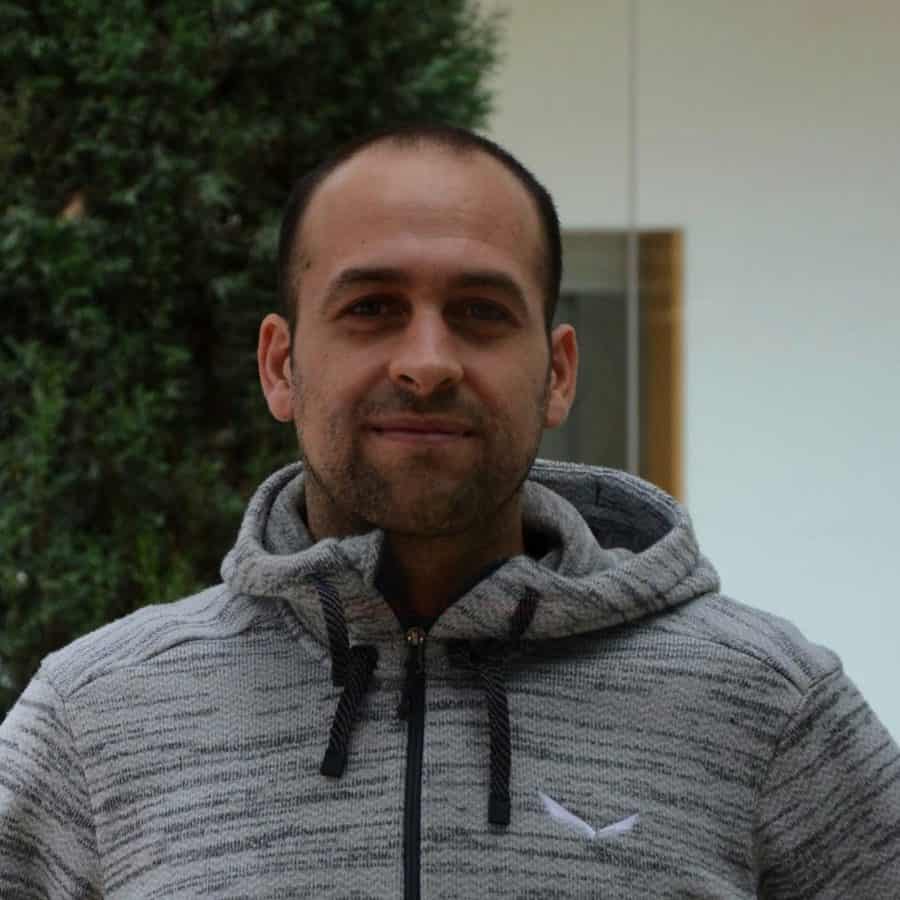
About the author:
Edgar Riba is a research scientist at IRI – Institut de Robòtica i Informàtica Industrial, CSIC-UPC. PhD candidate at the Computer Vision Center, Universitat Autònoma de Barcelona and Member of OpenCV.org Technical Committee. He is the creator of Kornia mentored by Gary Bradski and currently is the project leader managing the main library activities and core development.
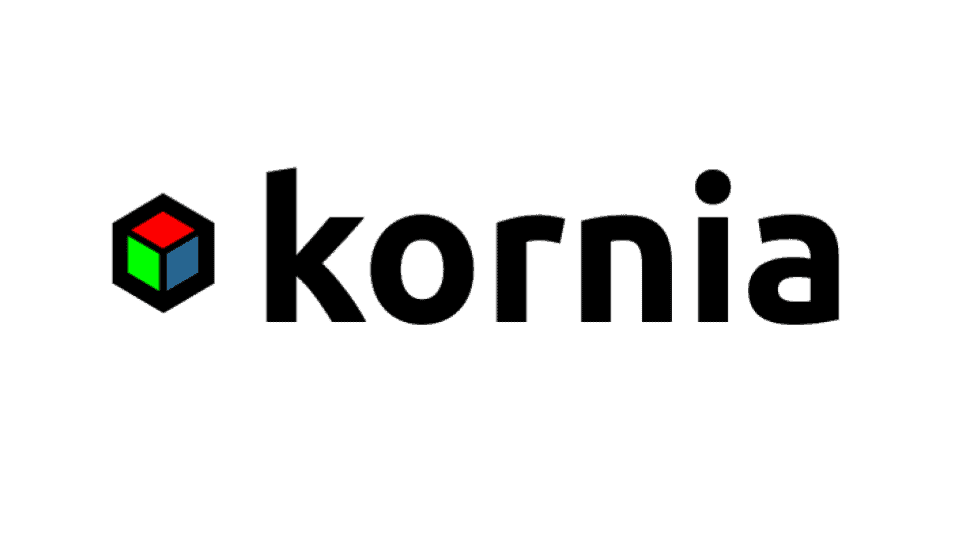
What is Kornia? Kornia [1, 2] can be defined as a computer vision library for PyTorch [3], inspired by OpenCV and with strong GPU support. Kornia allows users to write code as if they were using native PyTorch providing high-level interfaces to vision algorithms computed directly on tensors. In addition, some of the main PyTorch features are inherited by Kornia such as a high-performance environment with easy access to automatic differentiation, executing models on different devices (CPU, GPU or Tensor Processing Unit — TPU), parallel programming by default, communication primitives for multi-process parallelism across several computation nodes and code ready for production.
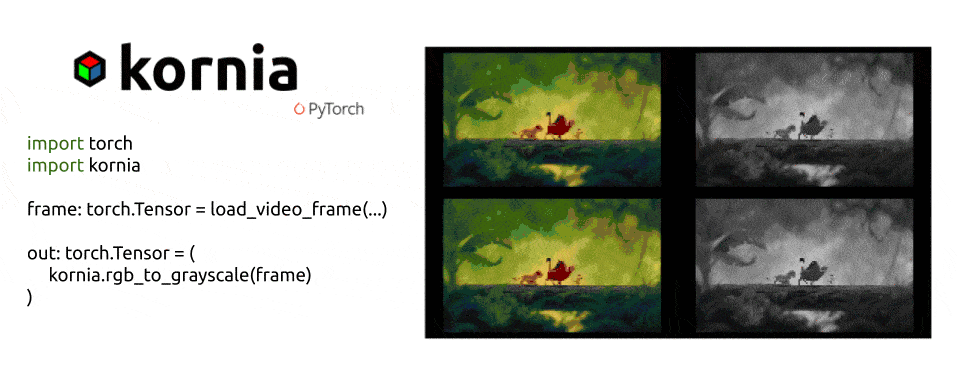
Differentiable. Any image processing algorithm that can be defined as a Direct Acyclic Graph (DAG) structure can be incorporated in a neural network and can be optimized during training, making use of the reverse-mode auto-differentiation, compute gradients via backpropagation. In practice, this means that computer vision functions are operators that can be placed as layers within the neural networks for training via backpropagating through them.
Transparent API. A key component in the library design is its easy way to seamlessly add hardware acceleration to your program with a minimum effort. The library API is agnostic to the input source device, meaning that the algorithms can either be executed in several device types such as CPU, GPU, or the recently introduced TPU.
Parallel programming. Batch processing is another important feature that enables to run vision operators using data parallelism by default. The assumption for the operators is to receive N-channel image tensors as input batches, contrary to standard vision libraries with single 1-3 channel images. Hence, working with multispectral, hyperspectral, or volumetric images can be done in a straight-forward manner using Kornia.
Distributed. Support for communication primitives for multi-process parallelism across several computation nodes running on one or more groups of local or cloud-based machines. The library design allows users to run their applications in different distributed systems, or even able to process large vision pipelines in an efficient way.
Production. Since version v1.0.0, PyTorch has the feature to serialize and optimize models for production purposes. Based on its just-in-time (JIT) compiler, PyTorch traces the models, creating TorchScript programs at runtime in order to be run in a standalone C++ program using kernel fusion to do faster inference. This makes our library a perfect fit also for built-in vision products.
Kornia aims to be a reimplementation for OpenCV for research purposes in the sense that mimics some of the main functionalities adding the ability to backpropagate through the different operators. However, note that Kornia does not seek to be a replacement for OpenCV since it is not optimized for production purposes or to achieve high-performance in embedded devices. Even though the project is backed up by OpenCV.org, there is no intention to merge in any form both projects in the mid-term. Kornia can be understood as a set of tools for training neural networks to be later used in production using other optimized frameworks.
Library structure
The internal structure of the library is designed to cover different computer vision areas, including color conversions, low-level image processing, geometric transformations, and some utilities for training such as specific loss functions, conversions between data layouts for different frameworks, or functionalities to easily visualize images and debug models during training.
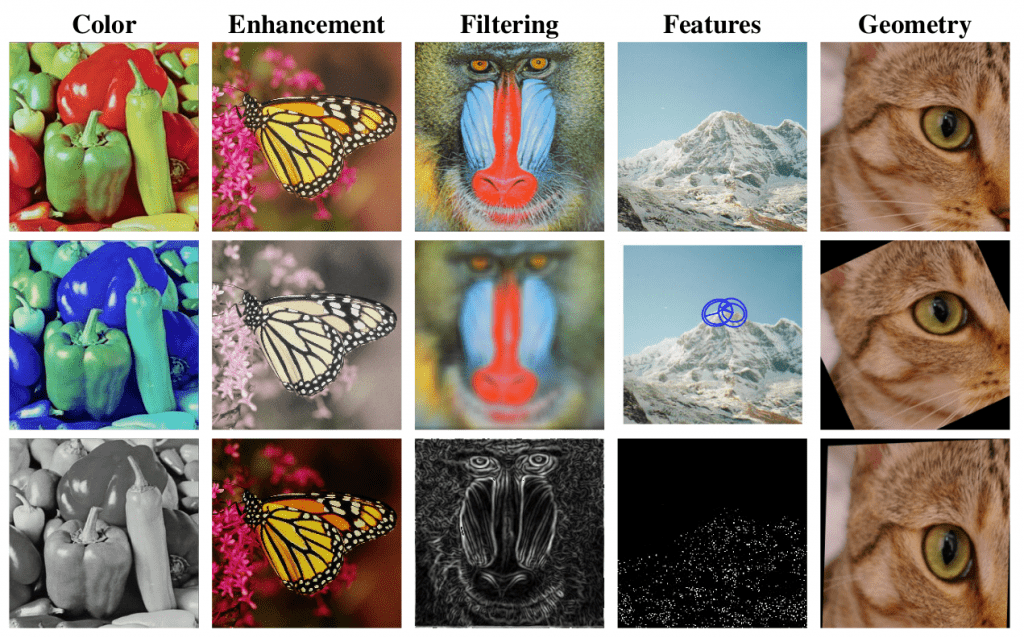
Component | Description |
kornia | a Differentiable Computer Vision library like OpenCV, with strong GPU support |
kornia.augmentation | a module to perform data augmentation in the GPU |
kornia.color | a set of routines to perform color space conversions |
kornia.contrib | a compilation of user contrib and experimental operators |
kornia.enhance | a module to perform normalization and intensity transformations |
kornia.feature | a module to perform feature detection |
kornia.filters | a module to perform image filtering and edge detection |
kornia.geometry | a geometric computer vision library to perform image transformations, 3D linear algebra and conversions using different camera models |
kornia.losses | a stack of loss functions to solve different vision tasks |
kornia.utils | image to tensor utilities and metrics for vision problems |
Getting started
Kornia is public available in GitHub [4] with an Apache License 2.0 and can be installed in any Linux, MacOS or Windows operating system, having PyTorch as a single dependency, through the Python Package Index (PyPI) using the following command:
pip install kornia
Start using Kornia along with OpenCV:
import cv2
import numpy as np
import torch
import kornia as K
img: np.ndarray = cv2.imread(“bruce.png”, cv2.IMREAD_COLOR) # HxWx3
img_t: torch.Tensor = K.image_to_tensor(img) # 1x3xHxW
Img_out: torch.Tensor = K.warp_perspective(img_t, H, (h, w)) # 1x3xHxW
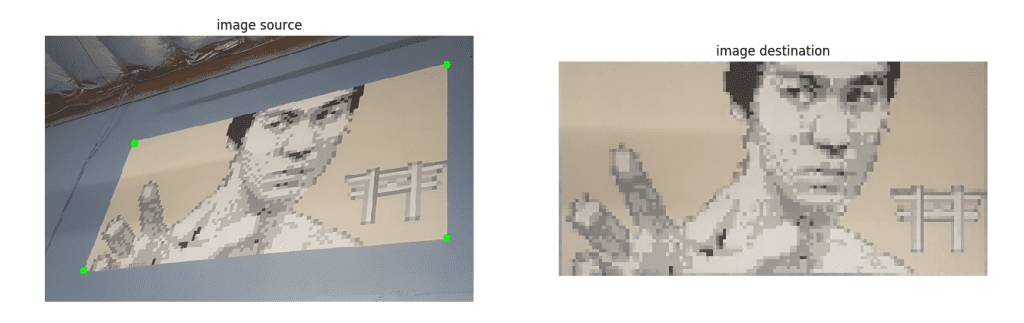
Kornia v0.4 release
Kornia team is happy to announce the release for v0.4.
We are releasing a new version for Kornia that includes different functionalities to work with 3D augmentations and volumetric data, local features matching, homographies and epipolar geometry.
In short, we list the following new features:
- Support for PyTorch v1.6.0.
- Local descriptors matching, homography and epipolar geometry API.
- 3D augmentations and low-level API to work with volumetric data.
Local features matching
We include an kornia.feature.matching API to perform local descriptors matching such classical and derived version of the nearest neighbor (NN).
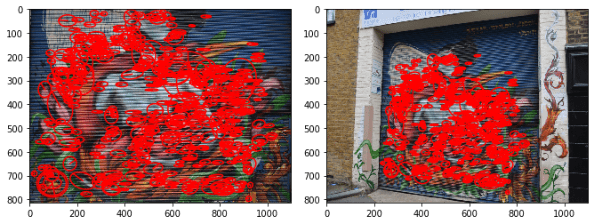
import torch
import kornia as K
desc1 = torch.rand(2500, 128)
desc2 = torch.rand(2500, 128)
dists, idxs = K.feature.matching.match_nn(desc1, desc2) # 2500 / 2500x2
Homography and epipolar geometry
We also introduce kornia.geometry.homography including different functionalities to work with homographies and differentiable estimators based on the DLT formulation and the iteratively-reweighted least squares (IRWLS).
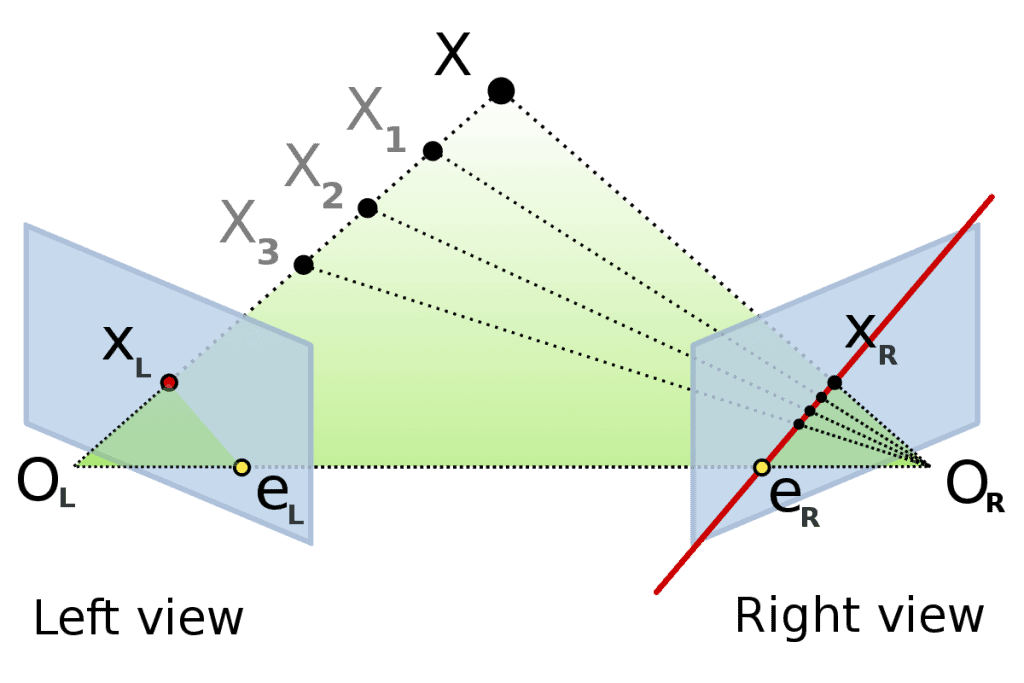
import torch
import kornia as K
pts1 = torch.rand(1, 8, 2)
pts2 = torch.rand(1, 8, 2)
H = K.find_homography_dlt(pts1, pts2, weights=torch.rand(1, 8)) # 1x3x3
In addition, we have ported some of the existing algorithms from opencv.sfm to PyTorch under kornia.geometry.epipolar that includes different functionalities to work with Fundamental, Essential or Projection matrices, and Triangulation methods useful for Structure from Motion problems.
3D augmentations and volumetric
We expand the kornia.augmentaion with a series of operators to perform 3D augmentations for volumetric data.
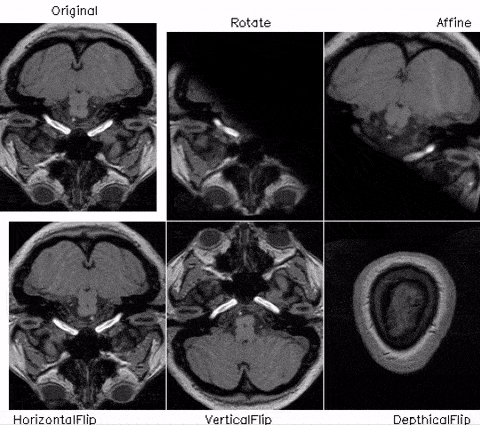
In this release, we include the following first set of geometric 3D augmentations methods:
- RandomDepthicalFlip3D (along depth axis)
- RandomVerticalFlip3D (along height axis)
- RandomHorizontalFlip3D (along width axis)
- RandomRotation3D
- RandomAffine3D
The API for 3D augmentation work same as with 2D image augmentations:
import torch
import kornia as K
x = torch.eye(3).repeat(3, 1, 1)
aug = K.augmentation.RandomVerticalFlip3D(p=1.0)
print(aug(x))
tensor([[[[[0., 0., 1.],
[0., 1., 0.],
[1., 0., 0.]],
<BLANKLINE>
[[0., 0., 1.],
[0., 1., 0.],
[1., 0., 0.]],
<BLANKLINE>
[[0., 0., 1.],
[0., 1., 0.],
[1., 0., 0.]]]]])
Finally, we also introduce a low level API to perform 4D features transformations kornia.warp_projective and extending the filtering operators to support 3D kernels kornia.filter3D.
More 2d operators
We expand as well the list of the 2D image augmentations based on the paper AutoAugment: Learning Augmentation Policies from Data.
- Solarize
- Posterize
- Sharpness
- Equalize
- RandomSolarize
- RandomPosterize
- RandomShaprness
- RandomEqualize
Please, do not hesitate to check the release notes on GitHub to learn about the new library features and get more details.
Have a happy coding day 🙂
#computervision #AI #deeplearning #pytorch
References
[1] Edgar Riba, Dmytro Mishkin, Dani Ponsa, Rublee Ethan, and Gary Bradski. Kornia: an open source differentiable computer vision library for pytorch. In Winter Conference on Applications of Computer Vision, 2020.
[2] Edgar Riba, Dmytro Mishkin, Jian Shi, Dani Ponsa, Francesc Moreno-Noguer, and Gary Bradski. A survey on Kornia: an open source differentiable computer vision library for pytorch. 2020.
[3] Adam Paszke, Sam Gross, Soumith Chintala, Gregory Chanan, Edward Yang, Zachary DeVito, Zeming Lin, Alban Desmaison, Luca Antiga, and Adam Lerer. Automatic differentiation in PyTorch. In NIPS-W, 2017.
[4] Kornia GitHub: https://github.com/kornia/kornia
5K+ Learners
Join Free VLM Bootcamp3 Hours of Learning